Table of Contents
Uncontrolled cloud costs pose an enormous risk for any organization. The longer these costs go ungoverned, the greater your risk. Volatile, unforeseen expenses eat into profits. Budgets become unstable. Waste and inefficiency go unchecked. Making strategic decisions becomes difficult, if not impossible. Uncertainty reigns.
Everybody’s cloud bill continues to get steeper month over month, and the most rapidly escalating (and often the single largest) slice of the pie is cloud costs for data analytics—usually at least 40%. With virtually every organization asking for ever more data analytics, and more data workloads moving to the cloud, uncontrolled cloud data costs increasingly become a bigger part of the overall problem.
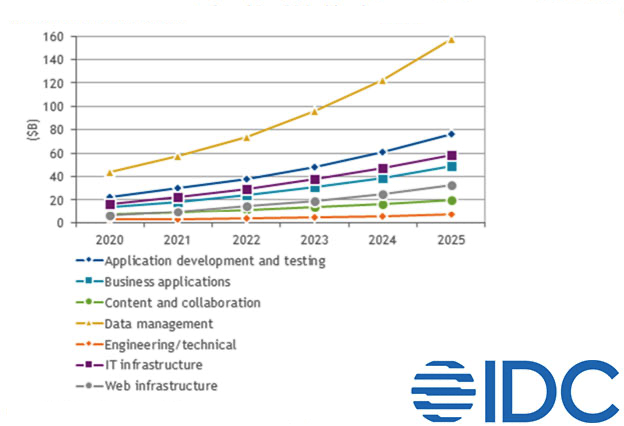
Data workloads are the fastest-growing cloud cost category and, if they’re not already, will soon be the #1 cloud expense.
All too often, business leaders don’t even have a clear understanding of where the money is going—or why—for cloud data expenditures, much less a game plan for bringing these costs under control.
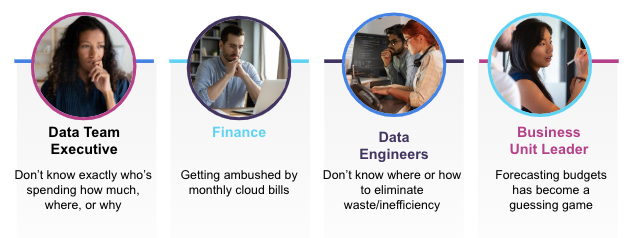
Ungoverned cloud data usage and costs result in multiple, usually coexisting, business vulnerabilities.
Consider these common scenarios:
- You’re a C-suite executive who is ultimately responsible for cloud costs, but can’t get a good explanation from your data team leaders about why your Azure Databricks or AWS or Google Cloud costs in particular are skyrocketing.
- You’re a data team leader who’s on the hook to explain to the C-suite these soaring cloud data costs, but can’t. You don’t have a good handle on exactly who’s spending how much on what. This is a universal problem, no matter what platform or cloud provider: 70% of organizations aren’t sure what they spend their cloud budget on.
- You’re in Finance and are getting ambushed by your AWS (or Databricks or GCP) bill every month. Across companies of every size and sector, usage and costs are wildly variable and unpredictable—one survey has found that cloud costs are higher than expected for 6 of every 10 organizations.
- You’re a business product owner who needs additional budget to meet the organization’s increasing demand for more data analytics but don’t really know how much more money you’ll need. Forecasting—whether for Snowflake, Databricks, Amazon EMR, BigQuery, or any other cloud service—becomes a guessing game: Forrester has found that 80% of companies have difficulty predicting data-related cloud costs, and it’s becoming ever more problematic to keep asking for more budget every 3-6 months.
- You’re an Engineering/Operations team lead who knows there’s waste and inefficiency in your cloud usage but don’t really know how much or exactly where, much less what to do about it. Your teams are groping in the dark, playing blind man’s bluff. And it’s getting worse: 75% of organizations report that cloud waste is increasing.
- Enterprise architecture teams are seeing their cloud migration and modernization initiatives stall out. It’s common for a project’s three-year budget to be blown by Q1 of Year 2. And increasingly companies are pulling the plug. A recent report states that 81% of IT teams have been directed by the C-suite to cut or halt cloud spending. You find yourself paralyzed, unable to move either forward or back—like being stranded in a canoe halfway through a river crossing.
- Data and Finance VPs don’t know the ROI of their modern data stack investments—or even how to figure that out. But whether you measure it or not, the ROI of your modern data stack investments nosedives as costs soar. You find it harder and harder to justify your decision to move to Databricks or Snowflake or Amazon EMR or BigQuery. PricewaterhouseCoopers has found that over half (53%) of enterprises have yet to realize substantial value from their cloud investments.
- With seemingly no end in sight to escalating cloud costs, data executives may even be considering the radical step of sacrificing agility and speed-to-market gains and going back to on-prem (repatriation). The inability to control costs is a leading reason why 71% of enterprises expect to move all or some of their workloads to on-prem environments.
How’d we get into this mess?
The problem of ungoverned cloud data costs is universal and has been with us for a while: 83% of enterprises cite managing cloud spend as their top cloud challenge, and optimizing cloud usage is the top cloud initiative for the sixth straight year, according to the 2022 State of the Cloud Report.
Some of that is simply due to the increased volume and velocity of data and analytics. In just a few short years, data analytics has gone from a science project to an integral business-critical function. More data workloads are running in the cloud, often more than anticipated. Gartner has noted that overall cloud usage for data workloads “almost always exceeds initial expectations,” stating that workloads may grow 4X or more in the first year alone.
If you’re like most data-driven enterprises, you’ve likely invested millions in the innovative data platforms and cloud offerings that make up your modern data stack—Databricks, Snowflake, Amazon EMR, BigQuery, Dataproc, etc.—and have realized greater agility and go-to-market speed. But those benefits have come at the expense of understanding and controlling the associated costs. You’re also seeing your cloud costs lurch unpredictably upwards month over month. And that’s the case no matter which cloud provider(s) you’re on, or which platform(s) you’re running. Your modern data stack consumes ever more budget, and it never seems to be enough. You’re under constant threat of your cloud data costs jumping 30-40% in just six months. It’s become a bit like the Wild West, where everybody is spinning up clusters and incurring costs left and right, but nobody is in control to govern what’s going on.
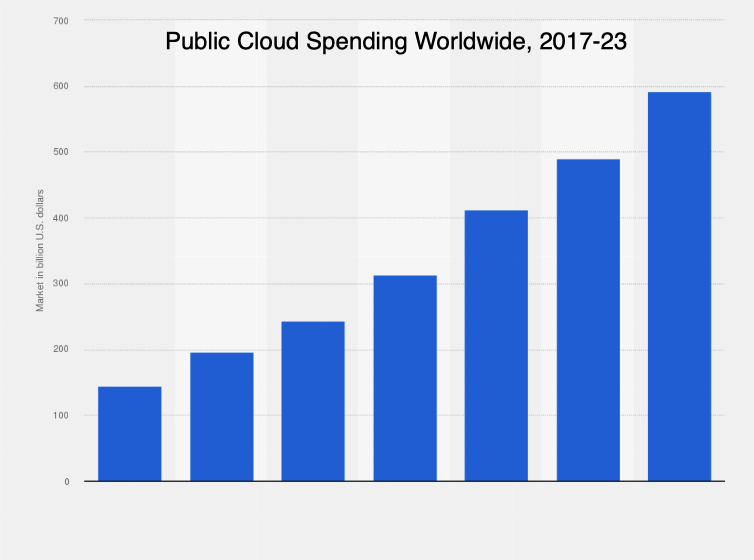
The only thing predictable about modern data stack costs is that they seem to always go up. Source: Statistica
Many organizations that have been wrestling with uncontrolled cloud data costs have begun adopting a FinOps approach. Yet they are struggling to put these commonsensical FinOps principles into practice for data teams. They find themselves hamstrung by generic FinOps tools and are hitting a brick wall when it comes to actualizing foundational core capabilities.
So why are organizations having trouble implementing DataFinOps (FinOps for data teams)?
FinOps for data teams
Just as DevOps and DataOps are “cousin” approaches—bringing agile and lean methodologies to software development and data management, tackling similar types of challenges, but needing very distinct types of information and analyses to get there—FinOps and DataFinOps are related but different. In much the same way (and for similar reasons) DevOps observability built for web applications doesn’t work for data pipelines and applications, DataFinOps brings FinOps best practices to data management, taking the best of FinOps to help data teams measure and improve cost effectiveness of data pipelines and data applications.
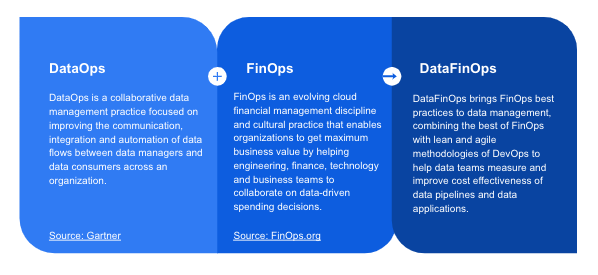
DataOps + FinOps = DataFinOps
FinOps principles and approach
As defined by the FinOps Foundation, FinOps is “an evolving cloud financial management discipline and cultural practice that enables organizations to get maximum business value by helping engineering, finance, technology and business teams to collaborate on data-driven spending decisions.”
It’s important to bear in mind that FinOps isn’t just about lowering your cloud bill—although you will wind up saving money in a lot of areas, running things more cost-effectively, and being able to do more with less (or at least the same). It’s about empowering engineers and business teams to make better choices about their cloud usage and deriving the most value from their cloud investments.
There are a few underlying “north star” principles that guide all FinOps activities:
- Cloud cost governance is a team sport. Too often controlling costs devolves into Us vs. Them friction, frustration, and finger-pointing. It can’t be done by just one group alone, working with their own set of data (and their own tools) from their own perspective. Finance, Engineering, Operations, technology teams, and business stakeholders all need to be on the same page, pulling in the same direction to the same destination, working together collaboratively.
- Spending decisions are driven by business value. Not all cloud usage is created equal. Not everything merits the same priority or same level of investment/expenditure. Value to the business is the guiding criterion for making collaborative and intelligent decisions about trade-offs between performance, quality, and cost.
- Everyone takes ownership of their cloud usage. Holding individuals accountable for their own cloud usage—and costs—essentially shifts budget responsibility left, onto the folks who actually incur the expenses.This is crucial to controlling cloud costs at scale, but to do so, you absolutely must empower engineers and Operations with the self-service optimization capabilities to “do the right thing” themselves quickly and easily.
- Reports are accessible and timely. To make data-driven decisions, you need accurate, real-time data. The various players collaborating on these decisions all bring their own wants and needs to the table, and everybody needs to be working from the same information, seeing the issue(s) the same way—a single pane of glass for a single source of truth.Dashboards and reports must be visible, understandable, and practical for a wide range of people making these day-to-day cost-optimization spending decisions.
Applying FinOps to data management
Data teams can put these FinOps principles into practice with a three-phase iterative DataFinOps life cycle:
- Observability, or visibility, is understanding what is going on in your environment, measuring everything, tracking costs, and identifying exactly where the money is going.
- Optimization is identifying where you can eliminate waste and inefficiencies, take advantage of less-expensive cloud pricing options, or otherwise run your cloud operations more cost-efficiently—and then empowering individuals to actually make improvements.
- Governance is all about going from reactive problem-solving to proactive problem-preventing, sustaining iterative improvements, and implementing guardrails and alerts.
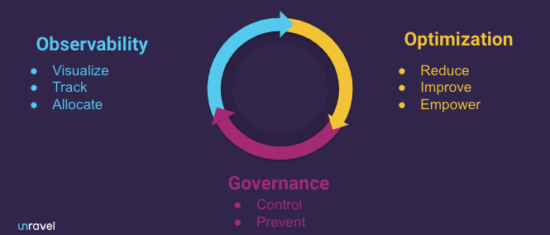
The DataFinOps life cycle: observability, optimization, and governance.
The essential principles and three-phase approach of FinOps are highly relevant to data teams. But just as DataOps requires a different set of information than DevOps, data teams need unique and data-specific details to achieve observability, optimization, and governance in their everyday DataFinOps practice.
What makes DataFinOps different—and difficult
The following challenges all manifest themselves in one way or another at each stage of the observability/optimization/governance DataFinOps life cycle. Be aware that while you can’t do anything until you have full visibility/observability into your cloud data costs, the optimization and governance phases are usually the most difficult and most valuable to put into action.
First off, just capturing and correlating the volume of information across today’s modern data stacks can be overwhelming. The sheer size and complexity of data applications/pipelines—all with multiple sub-jobs and sub-tasks processing in parallel—generates millions of metrics, metadata, events, logs, etc. Then everything has to be stitched together somehow in a way that makes sense to Finance and Data Engineering and DataOps and the business side.
Even a medium-sized data analytics operation has 100,000s of individual “data-driven spending decisions” to make. That’s the bad news. The good news is that this same complexity means there are myriad opportunities to optimize costs.
Second, the kinds of details (and the level of granularity) your teams need to make informed, intelligent DataFinOps decisions simply are not captured or visualized by cloud cost-management FinOps tools or platform-specific tools like AWS Cost Explorer, OverWatch, or dashboards and reports from GCP and Microsoft Cost Management. It’s the highly granular details about what’s actually going on in your data estate (performance, cost) that will uncover opportunities for cloud cost optimizations. But those granular details are scattered across dozens of different tools, technologies, and platforms.
Third, there’s usually no single source of truth. Finance, data engineers, DataOps, and the business product owners all use their own particular tools of choice to manage different aspects of cloud resources and spending. Without a common mechanism (the proverbial single pane of glass) to see and measure cost efficiency, it’s nearly impossible for any of them to make the right call.
Finally, you need to be able to see the woods for the trees. Seemingly simple, innocuous changes to a single cloud data application/pipeline can have a huge blast radius. They’re all highly connected and interdependent: the output of something upstream is the input to something else downstream or, quite likely, another application/pipeline for somebody somewhere else in the company. Everything must be understood within a holistic business context so that everybody involved in DataFinOps understands how everything is working as a whole.
Implementing DataFinOps in practice: A 6-step game plan
At its core, DataFinOps elevates cost as a first-class KPI metric, right alongside performance and quality. Most data team SLAs revolve around performance and quality: delivering reliable results on time, every time. But with cloud spend spiraling out of control, now cost must be added to reliability and speed as a co-equal consideration.
1. Set yourself up for success
Before launching DataFinOps into practice, you need to lay some groundwork around organizational alignment and expectations. The collaborative approach and the principle of holding individuals accountable for their cloud usage are a cultural sea-change. DataFinOps won’t work as a top-down mandate without buy-in from team members further down the ladder. Recognize that improvements will grow proportionally over time as your DataFinOps practice gains momentum. It’s best to adopt a crawl-walk-run approach, where you roll out a pilot project to discover best practices (and pitfalls) and get a quick win that demonstrates the benefits. Find a data analyst or engineer with an interest in the financial side who can be the flag-bearer, maybe take some FinOps training (the FinOps Foundation is an excellent place to start), and then dig into one of your more expensive workloads to see how to apply DataFinOps principles in practice. Similarly, get at least one person from the finance and business teams who is willing to get onboard and discover how DataFinOps works from their side.
Check out this 15-minute discussion on how JPMorgan Chase & Co, tackled FinOps at scale.
2. Get crystal-clear visibility into where the money is going
Before you can even begin to control your cloud costs, you have to understand—with clarity and precision—where the money is going. Most organizations have only hazy visibility into their overall cloud spend. The 2022 State of Cloud Cost Report states that gaining visibility into cloud usage is the single biggest challenge to controlling costs. The 2022 State of Cloud Cost Intelligence Report further finds that only 3 out of 10 organizations know exactly where their spend is going, with the majority either guesstimating or having no idea. And the larger the company, the more significant the cost visibility problem.
A big part of the problem are the cloud bills themselves. They’re either opaque or inscrutable; either way, they lack the business context that makes sense to your particular organization. Cloud vendor billing consoles (and third-party cost-management tools) give you only an aggregated view of total spend for different services (compute, storage, platform, etc.).
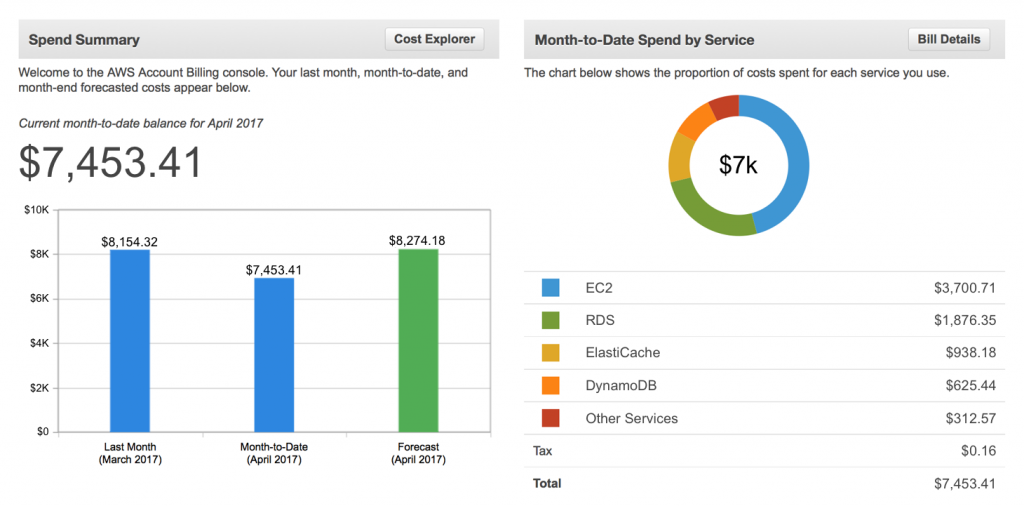
Cloud bills don’t answer questions like, Who spent on these services? Which department, teams, users are the top spenders?
Or you have to dedicate a highly trained expert or two to decode hundreds of thousands (millions, even) of individual billing line items and figure out who submitted which jobs (by department, team, individual user) for what purpose—who’s spending what, when, and why.
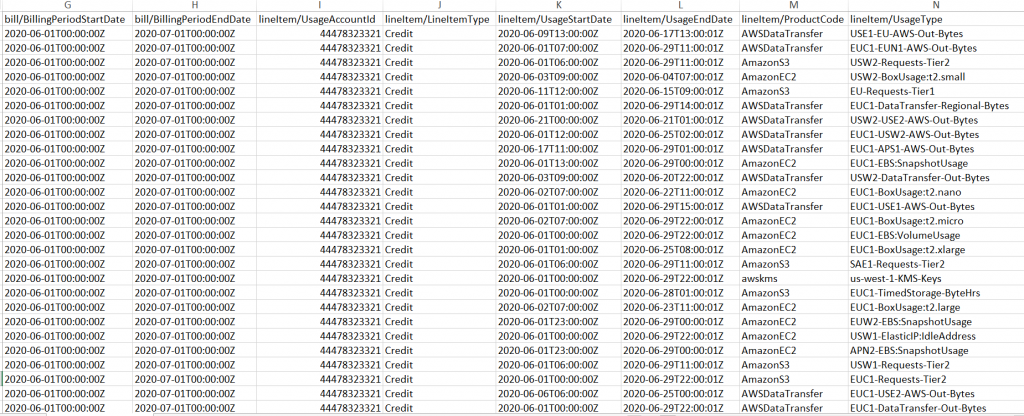
Decoding 1,000,000s of individual lines of billing data is a full-time job.
The lack of visibility is compounded by today’s heterogeneous multi-cloud, multi-platform reality. Most enterprise-scale organizations use a combination of different cloud providers and different data platforms, often the same platform on different cloud providers. While this is by no means a bad thing from an agility perspective, it does make comprehending overall costs across different providers even more difficult.
So, the first practical step to implementing DataFinOps is understanding your costs with clarity and precision. You need to be able to understand at a glance, in terms that make sense to you, which projects, applications, departments, teams, and individual users are spending exactly how much, and why.
You get precise visualization of cloud data costs through a combination of tagging and full-stack observability. Organizations need to apply some sort of cost-allocation tagging taxonomy to every piece of their data estate in order to categorize and track cloud usage. Then you need to capture a wide and deep range of granular performance details, down to the individual job level, along with cost information.
All this information is already available in different systems, hidden in plain sight. What you need is a way to pull it all together, correlating everything in a data model and mapping resources to the tagged applications, teams, individuals, etc.
Once you have captured and correlated all performance/cost details and have everything tagged, you can slice and dice the data to do a number of things with a high degree of accuracy:
- See who the “big spenders” are—which departments, teams, applications, users are consuming the most resources—so you can prioritize where to focus first on cost optimization, for the biggest impact.
- Track actual spend against budgets—again, by project or group or individual. Know ahead of time whether usage is projected to be on budget, is at risk, or has already gone over. Avoid “sticker shock” surprises when the monthly bill arrives.
- Forecast with accuracy and confidence. You can run regular reports that analyze historical usage and trends (e.g., peaks and valleys, auto-scaling) to predict future capacity needs. Base projections on data, not guesswork.
- Allocate costs with pinpoint precision. Generate cost-allocation reports that tell you exactly—down to the penny—who’s spending how much, where, and on what.
3. Understand why the costs are what they are
Beyond knowing what’s being spent and by whom, there’s the question of how money is being spent—specifically, what resources were allocated for the various workload jobs and how much they cost. Your data engineers and DataOps teams need to be able to drill down into the application- and cluster-level configuration details to identify which individuals are using cloud data resources, the size and number of resources they’re using, which jobs are being run, and what their performance looks like. For example, some data jobs are constrained by network bandwidth, while others are memory- or CPU-bound. Running those jobs on clusters and instance types may not maximize the return on your cloud data investments. If you think about cost optimization as a before-and-after exercise, your teams need a clear and comprehensive picture of the “before.”
With so many different cloud pricing models, instance types, platforms, and technologies available to choose from, you need everybody to have a fully transparent 360° view into the way expenses are being incurred at any given time so that the next step—cost optimization and collaborative decision-making about cloud spend—can happen intelligently.
4. Identify opportunities to do things more cost-effectively
If the observability phase gives you visibility into where costs are going, how, and why, the optimization phase is about taking that information and figuring out where you can eliminate waste, remove inefficiencies, and leverage different options that are less expensive but still meet business needs.
Most companies have very limited insight (if any) into where they’re spending more than they need to. Everyone knows on an abstract level that cloud waste is a big problem in their organization, but identifying concrete examples that can be remediated is another story. Various surveys and reports peg the amount of cloud budget going to waste between 30-50%, but the true percentage may well be higher—especially for data teams—because most companies are just guessing and tend to underestimate their waste. For example, after implementing cloud data cost optimization, most Unravel customers have seen their cloud spend lowered by 50-60%
An enterprise running 10,000s (or 100,000s) of data jobs every month has literally millions of decisions to make—at the application, pipeline, and cluster level—about where, when, and how to run those jobs. And each individual decision about resources carries a price tag.
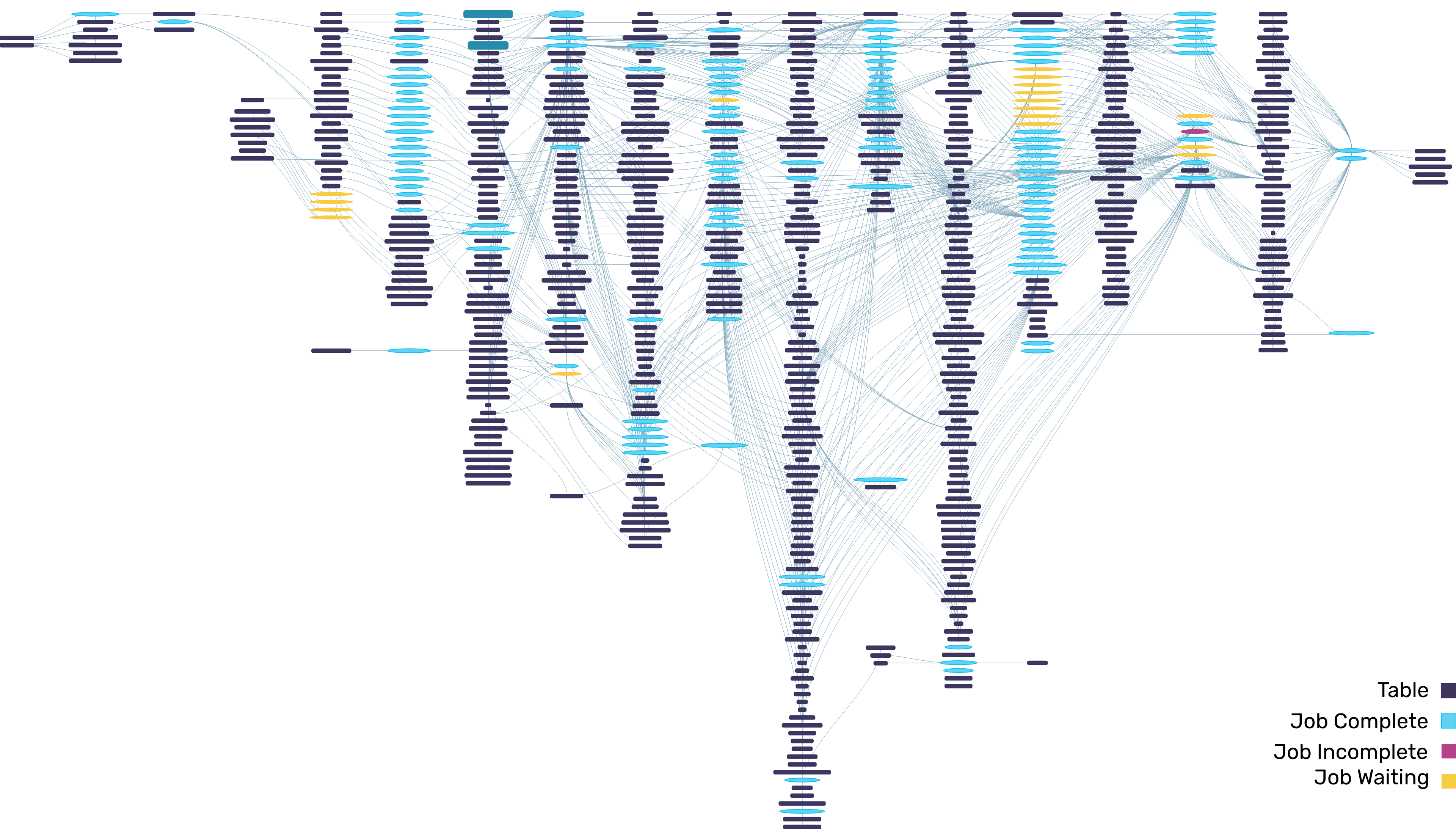
Enterprises have 100,000s of places where cloud data spending decisions need to be made.
Only about 10% of cost-optimization opportunities are easy to see, for example, shutting down idle clusters (deployed but no-longer-in-use resources). The remaining 90% lie below the surface.
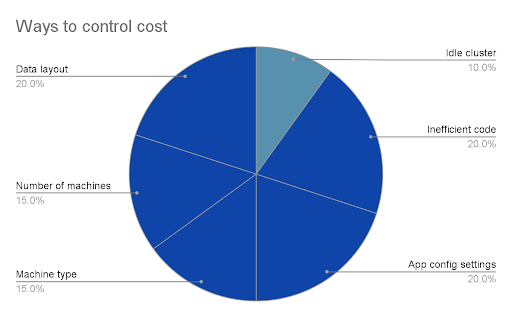
90% of cloud data cost-optimization opportunities are buried deep, out of immediate sight.
You need to go beyond knowing how much you are currently spending, to knowing how much you should be spending in a cost-optimized environment. You need insight into what resources are actually needed to run the different applications/pipelines vs. what resources are being used.
Data engineers are not wasting money intentionally; they simply don’t have the insights to run their jobs most cost-efficiently. Identifying waste and inefficiencies needs two things in already short supply: time and expertise. Usually you need to pull engineering or DataOps superstars away from what they’re doing—often the most complex or business-critical projects—to do the detective work of analyzing usage vs. actual need. This kind of cost-optimization analysis does get done today, here and there, on an ad hoc basis for a handful of applications, but doing so at enterprise scale is something that remains out of reach for most companies.
The complexity is overwhelming: for example, AWS alone has more than 200 cloud services and over 600 instance types available, and has changed its prices 107 times since its launch in 2006. For even your top experts, this can be laborious, time-consuming work, with continual trial-and-error, hit-or-miss attempts.
Spoiler alert: You need AI
AI is crucial to understanding where cost optimization is needed—at least, at enterprise scale and to make an impact. It can take hours, days, sometimes weeks, for even your best people to tune a single application for cost efficiency. AI can do all this analysis automatically by “throwing some math” at all the observability performance and cost data to uncover where there’s waste and inefficiencies.
- Overprovisioned resources. This is most often why budgets go off the rails, and the primary source of waste. The size and number of instances and containers is greater than necessary, having been allocated based on perceived need rather than actual usage. Right-sizing cloud resources alone can save an organization 50-60% of its cloud bill.
- Instance pricing options. If you understand what resources are needed to run a particular application (how that application interacts with other applications), the DataFinOps team can make informed decisions about when to use on-demand, reserved, or spot instances. Leveraging spot instances can be up to 90% less expensive than on-demand—but you have to have the insights to know which jobs are good candidates.
- Bad code. AI understands what the application is trying to do and can tell you that it’s been submitted in a way that’s not efficient. We’ve seen how a single bad join on a multi-petabyte table kept a job running all weekend and wound up costing the company over $500,000.
- Intelligent auto-scaling. The cloud’s elasticity is great but comes at a cost, and isn’t always needed. AI analyzes usage trends to help predict when auto-scaling is appropriate—or when rescheduling the job may be a more cost-effective option.
- Data tiering. You probably have petabytes of data. But you’re not using all of it all of the time. AI can tell you which datasets are (not) being used, applying cold/warm/hot labels based on age or usage, so you understand which ones haven’t been touched in months yet still sit on expensive storage. Moving cold data to less-expensive options can save 80-90% on storage costs.
5. Empower individuals with self-service optimization
Holding individual users accountable for their own cloud usage and cost is a bedrock principle of DataFinOps. But to do that, you also have to give them the insights and actionable intelligence for them to make better choices.
You have to make it easy for them to do the right thing. They can’t just be thrown a bunch or charts and graphs and metrics, and be expected to figure out what to do. They need quick and easy-to-understand prescriptive recommendations on exactly how to run their applications reliably, quickly, and cost-efficiently.
Ideally, this is where AI can also help. Taking the analyses of what’s really needed vs. what’s been requested, AI could generate advice on exactly where and how to change settings or configurations or code to optimize for cost.
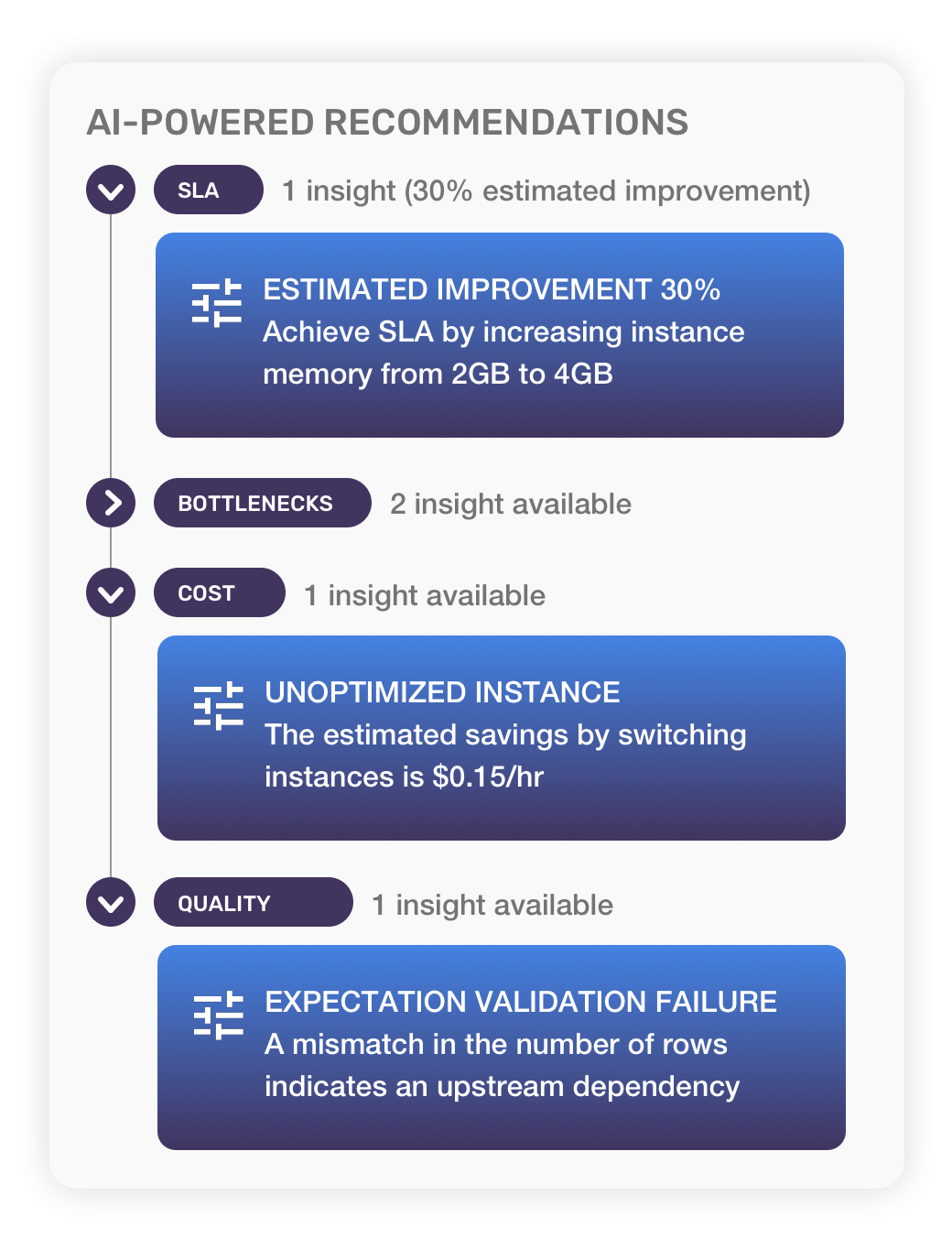
Leveraging millions of data points and hundreds of cost and performance algorithms, AI offers precise, prescriptive recommendations for optimizing costs throughout the modern data stack.
6. Go from reactive to proactive
Optimizing costs reactively is of course highly beneficial—and desperately needed when costs are out of control—but even better is actively governing them. DataFinOps is not a straight line, with a beginning and end, but rather a circular life cycle. Observability propels optimization, whose outcomes become baselines for proactive governance and feed back into observability.
Governance is all about getting ahead of cost issues beforehand rather than after the fact. Data team leaders should implement automated guardrails that give a heads-up when thresholds for any business dimension (projected budget overruns, jobs that exceed a certain size, time, cost) are crossed. Alerts could be triggered whenever a guardrails constraint is violated, notifying the individual user—or sent up the chain of command—that their job will miss its SLA or cost too much money and they need to find less expensive options, rewrite it to be more efficient, reschedule it, etc. Or guardrail violations could trigger preemptive corrective “circuit breaker’ actions to kill jobs or applications altogether, request configuration changes, etc., to rein in rogue users, put the brakes on runaways jobs, and nip cost overruns in the bud.
Controlling particular users, apps, or business units from exceeding certain behaviors has a profound impact on reining in cloud spend.
Conclusion
The volatility, obscurity, and lack of governance over rapidly growing cloud data costs introduce a high degree of unpredictability—and risk—into your organization’s data and analytics operations. Expenses must be brought under control, but reducing costs by halting activities can actually increase business risks in the form of lost revenue, SLA breaches, or even brand and reputation damage. Taking the radical step of going back on-prem may restore control over costs but sacrifices agility and speed-to-market, which also introduces the risk of losing competitive edge.
A better approach, DataFinOps, is to use your cloud data spend more intelligently and effectively. Make sure that your cloud investments are providing business value, that you’re getting the highest possible ROI from your modern data stack. Eliminate inefficiency, stop the rampant waste, and make business-driven decisions—based on real-time data, not guesstimates—about the best way to run your workloads in the cloud.
That’s the driving force behind FinOps for data teams, DataFinOps. Collaboration between engineering, DataOps, finance, and business teams. Holding individual users accountable for their cloud usage (and costs).
But putting DataFinOps principles into practice is a big cultural and organizational shift. Without the right DataFinOps tools, you’ll find it tough sledding to understand exactly where your cloud data spend is going (by whom and why) and identify opportunities to operate more cost-efficiently. And you’ll need AI to help empower individuals to optimize for cost themselves.
Then you can regain control over your cloud data costs, restore stability and predictability to cloud budgets, be able to make strategic investments with confidence, and drastically reduce business risk.